AI detectors work by examining the perplexity and burstiness of your text to determine if it’s human or machine-generated. The term perplexity refers to how predictable your text is. Typically, AI displays a lower level of perplexity because of its predictable nature.
Burstiness, on the other hand, assesses the variety in your sentence structures. AI’s uniformity in this aspect can often betray its non-human source. These tools depend on such metrics to distinguish between human and AI-generated content.
Nevertheless, advanced AI models like GPT-3 and GPT-4 are challenging these detectors as they blur the distinctions. Knowing how these detectors operate can provide valuable insights into the ongoing struggle between human creativity and AI imitation.
Table of Contents
Key Points
- AI detectors work by analyzing text. They use a method called perplexity to measure how predictable the text is.
- They also look at something called burstiness, which is a way to measure how variable the sentence structures are.
- Typically, text that has low perplexity and less burstiness is often written by AI.
- There are some limitations to these detectors. One of them is the constant improvement in AI capabilities. Another is the difficulty of dealing with complex grammar.
- To keep up with these challenges, it’s necessary to regularly update these detectors. This helps them accurately detect content written by new AI models.
Understanding AI Detectors
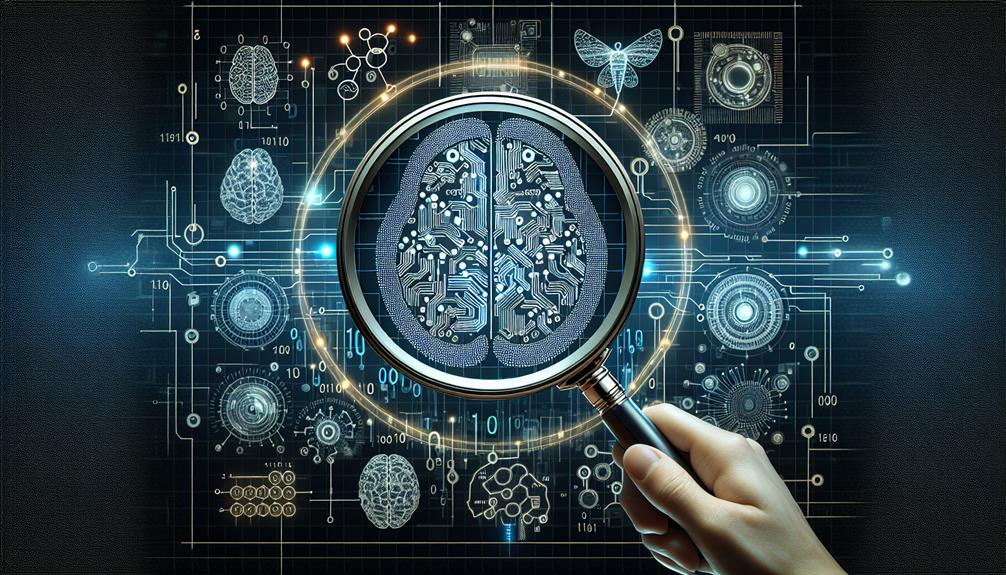
AI detectors use two key metrics, perplexity and burstiness, to differentiate between AI-generated and human writing. The former, perplexity, looks at how predictable or orderly the text is. AI-written texts usually have lower perplexity, meaning they follow a stricter pattern compared to human writing that often includes a wider range of sentence structures.
Burstiness, on the other hand, looks at how sentence structures and lengths vary in a piece of writing. Texts produced by AI typically show less burstiness, meaning they’re more uniform and less varied, unlike human writing, which tends to be more vibrant and changeable.
These metrics are vital tools for identifying AI-generated content. They give us a deep look into the text’s logical flow, level of predictability, and variety in sentence structure, which all together allow for a thorough, technical examination of AI writing.
Accuracy and Limitations
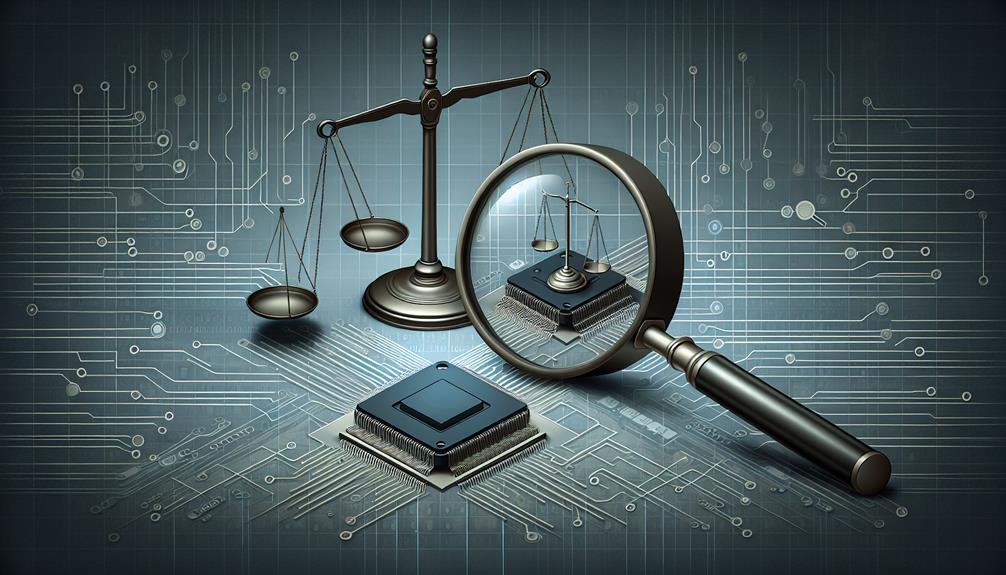
The rapid evolution of AI technologies like GPT-3 and GPT-4 is making AI-generated content more nuanced and varied, much like human-written text. This sophistication tests the accuracy of these detectors.
One of the key challenges these detectors face is in analyzing texts with complex grammar and spelling. These aspects greatly affect the assessment scores, making it difficult for the detectors to distinguish between AI and human outputs. Therefore, the need for these detectors to evolve is clear. They need to keep pace with the technologies they’re designed to monitor and adapt to the increasingly indistinguishable boundaries between AI and human-produced texts.
Despite the limitations, AI detectors continue to play a crucial role in distinguishing AI-generated text from human-created content.
Detection in Practice
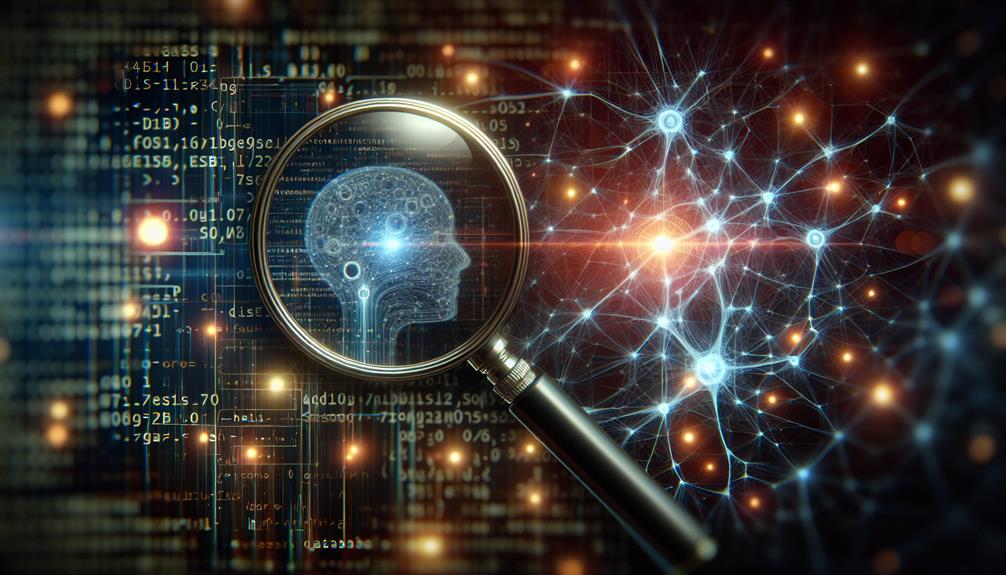
Using AI detectors can be quite handy. They work by examining the language in a text to determine if it’s written by a person or an AI. The key here is to understand how to read the metrics these tools provide.
For example, low perplexity often signifies AI-written text, while reduced burstiness indicates less variation in sentences, suggesting it’s not human-like. Once you get a grasp on these concepts, it becomes easier to tell the difference between human and AI-produced content.
In practice, this involves using AI detectors to analyze texts. These tools provide probabilities about the origin of the text. You can then use this information to judge whether a piece of content was created with AI tools. Using this technical approach can help accurately differentiate between content that’s been generated by an AI and content that’s been written by a human.
Future Developments
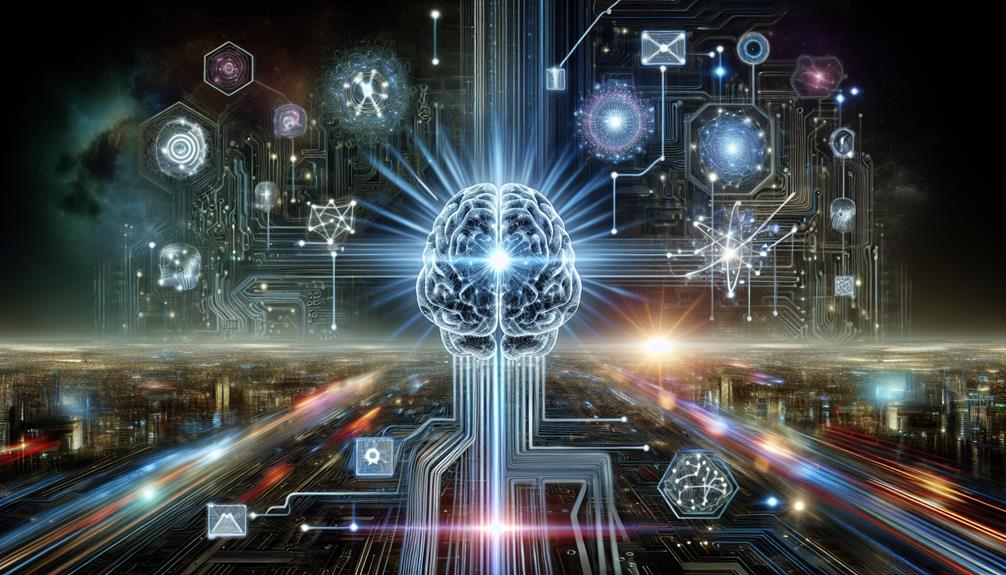
Looking at what the future holds, OpenAI is researching the use of embedded watermarks in texts generated by artificial intelligence as a way to confirm where they came from. This represents a significant advancement in the process of separating human- and machine-generated content. Questions still surround how effective and enduring these watermarks will be, but there’s no doubt they could redefine the tools used for AI detection.
Watermarks might provide a reliable way to identify AI-created content, which would significantly improve the reliability and accuracy of AI detectors. As this research moves forward, the use of watermarks in AI detection methods could provide solutions to some of the major issues surrounding the authenticity of digital content.
This growing field of study further emphasizes the need for ongoing innovation in AI detection.
Conclusion
AI detectors are like experienced investigators, examining details with a level of accuracy often exceeding human abilities. Yet, they’re not perfect.
Consider the task of finding a single piece of data in a vast digital database. AI can accomplish this incredibly quickly, showing theire improving precision, which now exceeds 90% in some fields. But their job doesn’t stop there.
As technology advances, AI will also improve in distinguishing real from fake, making the future of detection a constantly changing mix of data.
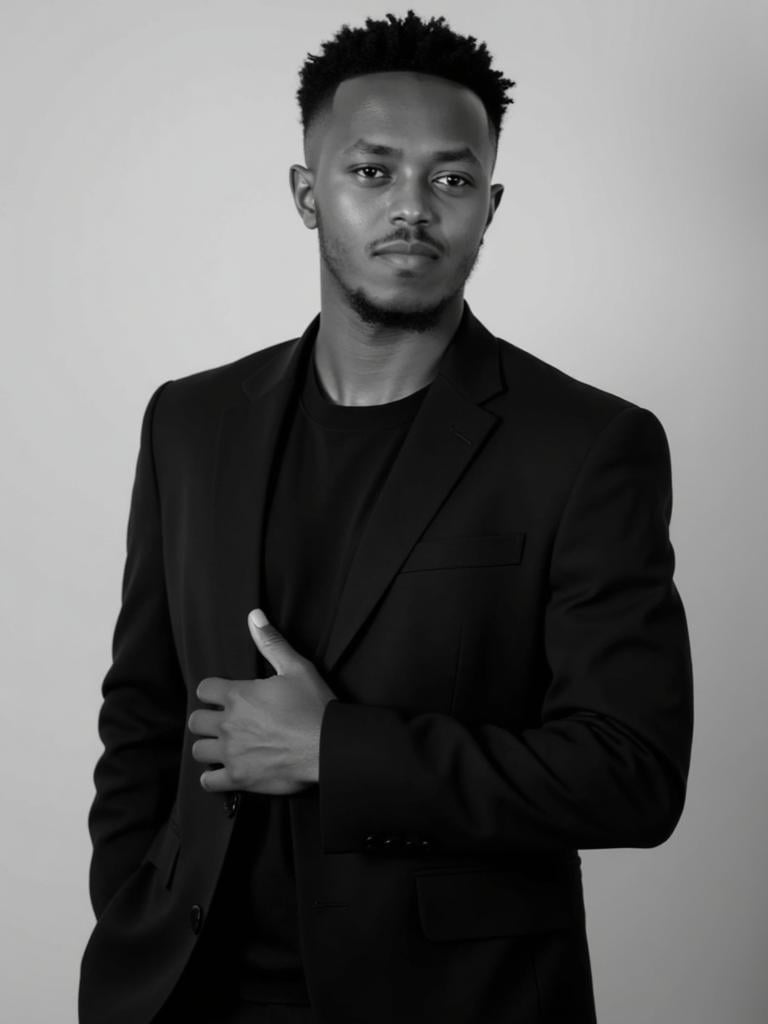
Paul Kinyua is a seasoned content writer with a passion for crafting engaging and informative tech and AI articles. With a knack for storytelling and a keen eye for detail, he has established himself as an authority in the field.